As the machine learning and artificial intelligence world keeps getting bigger with larger datasets, the requirement of better processors to be able to efficiently process and infer them is gaining equal importance. But, this deep learning computing cannot be achieved with general purpose chips. Therefore, along with giant companies like NVIDIA and Intel, startups are also getting into the space to boost production.
In 2022, the hardware industry witnessed massive leaps in the capabilities of the chips as well as the development of supercomputers.
Check out this list of top AI hardware released in 2022!

In March, NVIDIA announced the release of their new DGX Station, DGX-1, and DGX-2 built on Volta GPU architecture. These AI supercomputers are built for deep learning training, accelerated analytics and inference.

The system includes the DGX A100, the flagship chip of NVIDIA designed for data centres. The chip has integrated eight GPUs and has a GPU memory of 640 GB.
In May, Intel’s Habana Labs launched the second generation of their deep learning processors for training and inference—Habana Gaudi2, alongside Habana Greco. Both the processors are built for AI deep learning applications and designed with 7nm technology.

The research lab said that their chips performed at double speed when compared to NVIDIA’s A100-80GB GPU.
In April, IBM launched their first Telum Processor-based system, IBM z16, after three years of production. Designed specifically for improving performance and efficiency for large datasets, the processor features on-chip acceleration for AI inference.
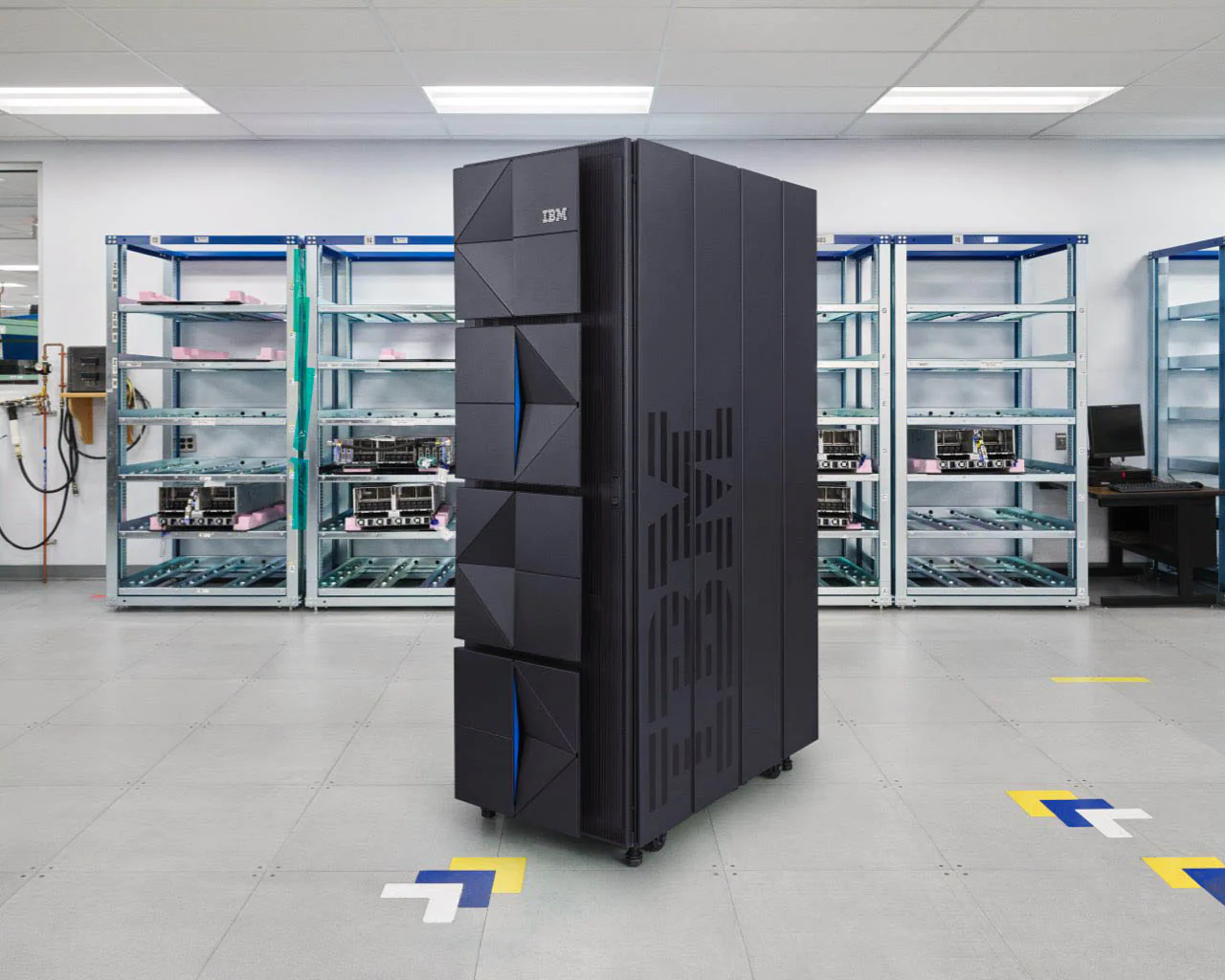
The platform brings AI and cyber resiliency for hybrid cloud working and utilises their quantum-safe technology.
In September, Advanced Micro Devices (AMD) released a newer version of their Zen microarchitecture—Zen 4—built on a 5 nm architecture.

Although AMD hasn’t been very vocal about developing hardware specifically for AI, the company introduced Ryzen 7000 series in May—a new line of PC processors that are built for machine learning capabilities—and expect to further grow with Zen 4.
Tesla Dojo Supercomputer
On the Tesla AI Day, apart from the Optimus Robot, Elon Musk also revealed the Dojo chip for faster training and inference in self-driving cars. Tesla claims that the four cabinets of Dojo chips can auto-label the same amount as 4,000 GPUs can label in 72 racks.
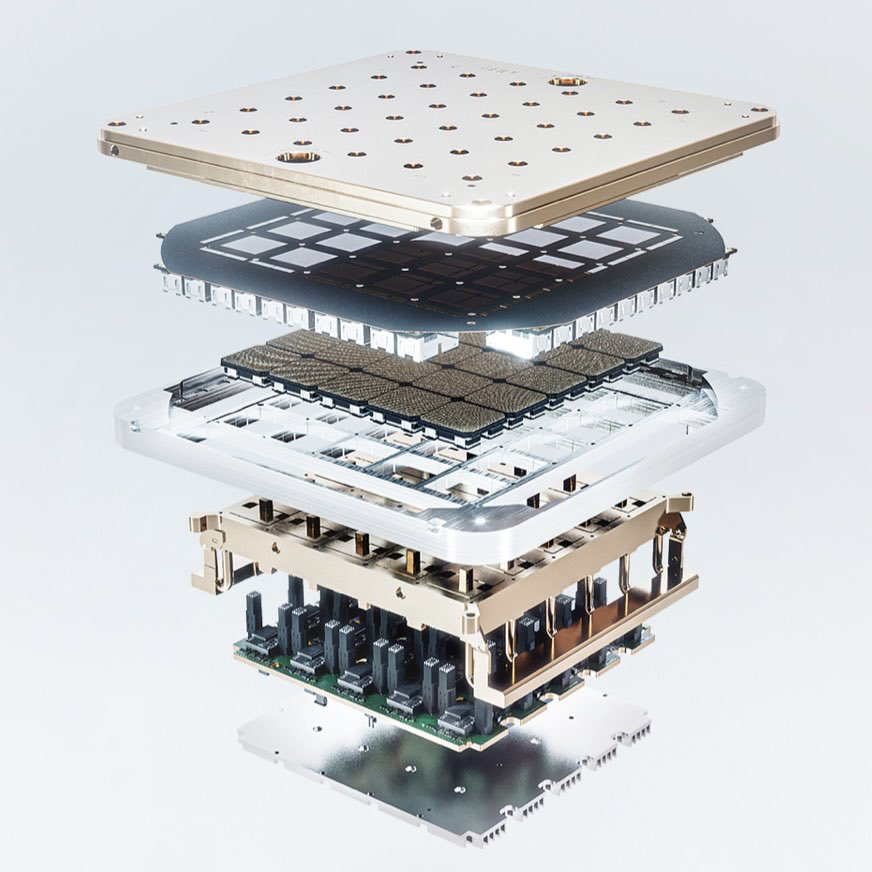
Previously, Tesla was using 5,760 NVIDIA A100 graphics cards for building FSD models in 720 nodes of eight GPUs. Apart from self-driving cars, the Dojo chip is also used in the Optimus Robot.
In November, Cerebras Systems introduced their AI supercomputer—Andromeda, built specifically for academic and commercial research by combining 16 Cerebras CS-2 systems. The company is majorly known for their dinner-plate-sized Wafer Scale Engine Two (WSE2) chips.
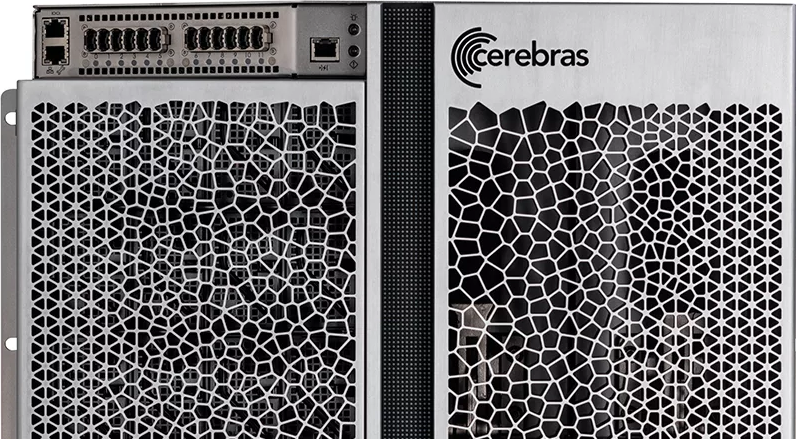
Andromeda can perform one quintillion operations per second or one exaflop AI computing based on 16-bit floating point format.
In September, SambaNova Systems announced that they will start shipping the second generation of the DataScale system—SN30. The system is powered by Cardinal SN30 chip and is built for large models with more than 100 billion parameters along with 2D and 3D images.
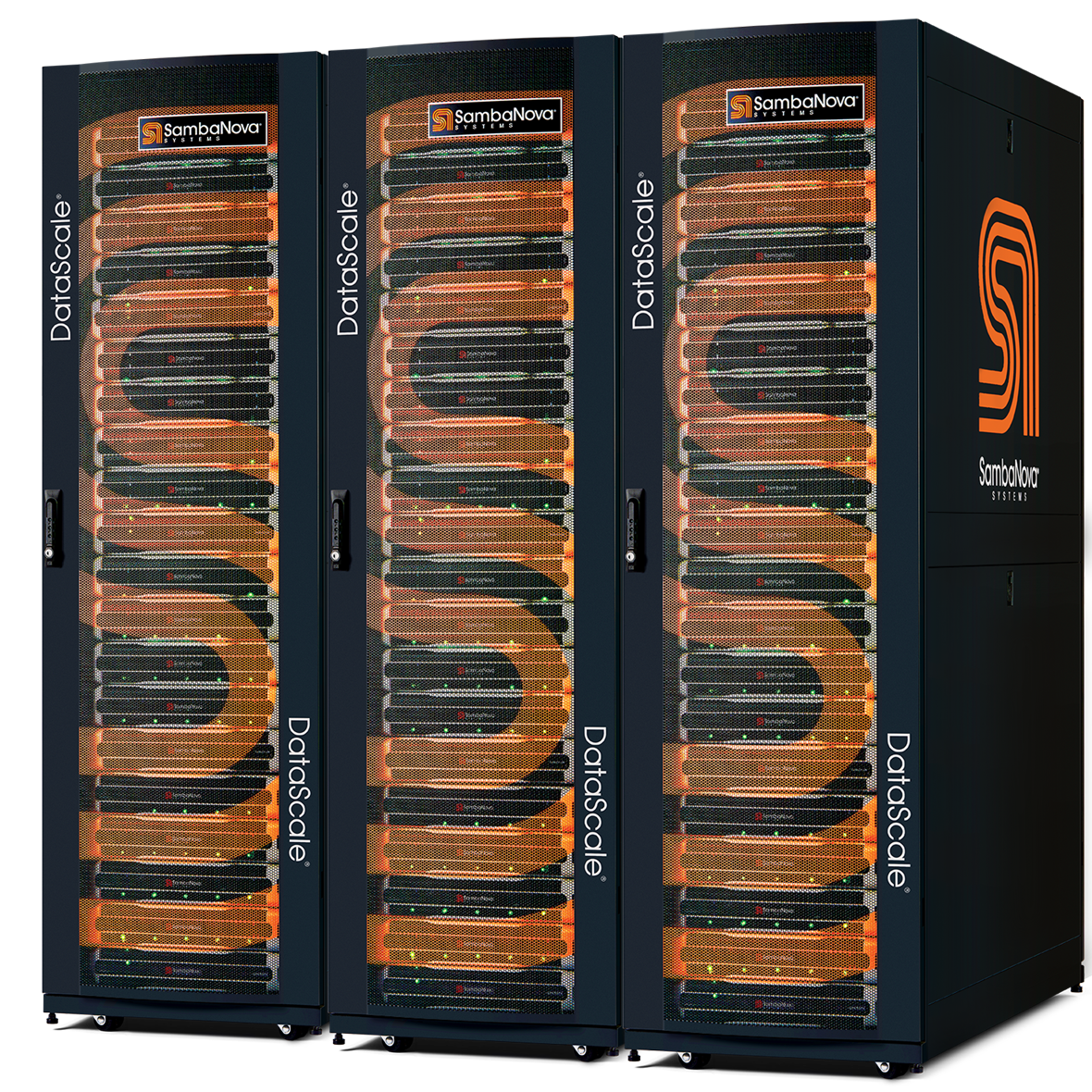
The fully integrated hardware and software system is built for AI and deep learning workloads with the chip made on TSMC’s 7nm process, capable of 688 teraflops. According to the company, the system offers 12.8X more memory capacity than NVIDIA’s DGX A100.